
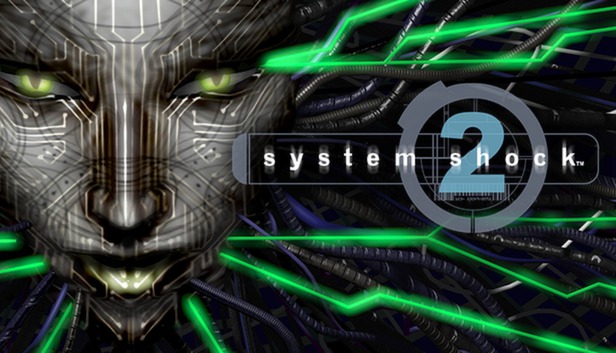
In addition, unexpected faults may happen due to an abrupt change in the environmental conditions including extreme weather events as well as performance degradation. In practice it is not easy to perform regular inspections for the maintenance of wind turbines that are located at inaccessible places, such as mountaintops, shorelines, or oceans, and deserts. Concretely, the maintenance cost accounts for more than 25% of the total cost. A survey on the total cost of wind power generation cost concluded that the operating cost, which includes maintenance (O&M), training operators and engineers, repair, system upgrades, inventory etc., is larger than capital costs, such as facility design, development planning, and construction. Wind turbines are one of the most remarkable renewable energy generation systems and many studies have been being conducted to reduce their operating cost. Finally, it was demonstrated that the proposed HMM algorithm achieved a greater than 95% detection success rate by using the long-term signals. A Hidden Markov model (HMM) is employed to propose the statistical fault detection algorithm in the time domain and the method whereby the input sequence for HMM is extracted is also introduced by considering the threshold levels and the correlation between the signals. The vibration distribution at a specific rotating speed of main shaft is approximated by the Weibull distribution and its cumulative distribution function is utilized for determining the threshold levels that indicate impending failure of mechanical parts. To this end, long-term vibration data were collected over two years by a CMS installed on a 3 MW wind turbine. The purpose of this study is to propose a fault detection algorithm for the mechanical parts of the wind turbine. For this reason, condition monitoring systems (CMSs) are typically installed to monitor their health condition.
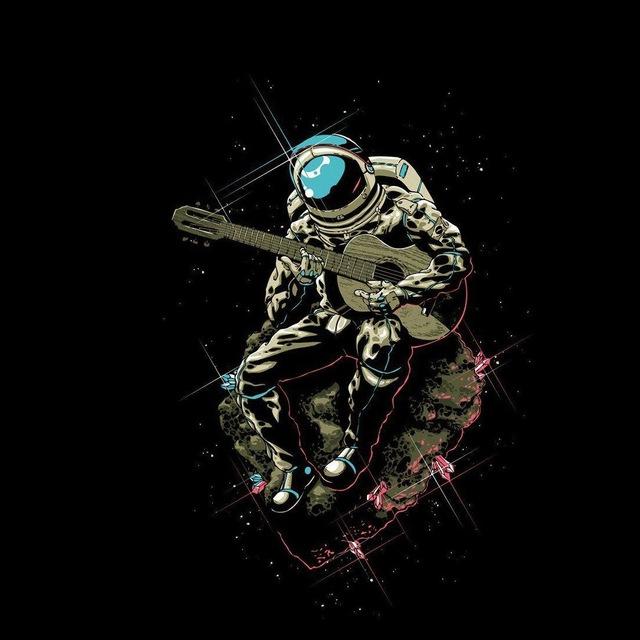
Regular inspection for the maintenance of the wind turbines is difficult because of their remote locations.
